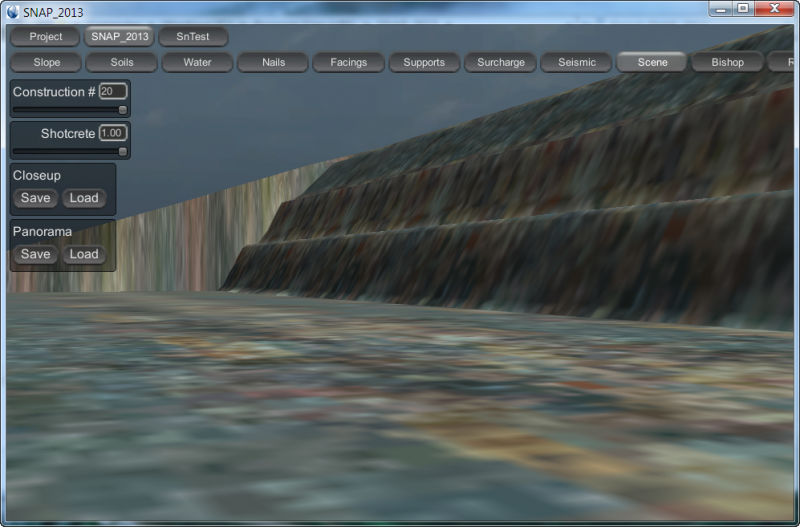
دانلود نرم افزار SNAP 2013 نسخه فول PREMIUM
SNAP می تواند به طرح دیوار ناخن خاک نزدیک به بهینه در دقیقه مطلوب به دست آوردن. این برنامه می تواند تنظیم:
دیوار
قد و خمیر زاویه
ناخن
طول
زاویه تمایل
زاویه فن
فاصله افقی و عمودی
فاصله های کنسول
اندازه نوار
مته قطر سوراخ
کاهش Backslope و کاهش downslope
کاربر محدوده معتبر و قطعنامه برای هر پارامتر، و همچنین به عنوان عوامل حداقل های ایمنی برای بارگذاری لرزه ای و بارگذاری شخص در هر برش مرحله بندی مشخص می کند.
VE طراحی به حداقل می رساند هزینه کل در حالی که جلسه تمام عوامل ایمنی ثبات جهانی است. طرح VE با استفاده از روش علمی به دست آمده. برنامه:
مشاهدات جمع آوری
ادعا روابط علی و نظریه پیشنهاد
تولید یک فرضیه
پیش بینی نتایج
اجرا می شود یک آزمایش برای به دست آوردن اندازه گیری
مقایسه پیش بینی های به اندازه گیری
دوباره ارزیابی نظریه ها، در صورت لزوم
تولید یک فرضیه جدید
این روند ادامه می یابد تا زمانی که نظریه های ارائه شده دقت پیش بینی هر گونه طراحی شده است.
روش هوش مصنوعی ترکیب شبکه های عصبی تخصصی استفاده می شود به مطالعه مشاهدات، تجزیه و تحلیل پارامترهای، پیشنهاد نظریهها، تولید فرضیه و پیش بینی نتایج.شبکه های عصبی شبیه سازی ساختار و عملکرد مغز می شود. این است که در مجموعه ای از مشاهدات (طرح دیوار شبیه سازی شده) برای پیش بینی نتایج حاصل از آزمایش های آینده (طراحی دیوار شبیه سازی شده) با تجزیه و تحلیل روابط علی و تعامل میان پارامترهای آموزش داده است.
شبکه های عصبی می رود از طریق یک فرآیند استدلال منطقی و فکر را به حدس تحصیل، یا فرضیه، از طراحی بهینه دیوار.شبکه های عصبی پیش بینی قیمت و حداقل عامل ایمنی این طراحی دیوار. این برنامه پس از محاسبه هزینه و ضریب اطمینان برای این طراحی دیوار. اگر پیش بینی شبکه عصبی نادرست است، شبکه های عصبی دوباره تجزیه و تحلیل روابط پارامتر برای تولید یک فرضیه جدید برای بهترین طراحی دیوار. تکرار این فرایند را تا زمانی که پیش بینی شبکه عصبی فرضیه درست است.
پس از آن شبکه های عصبی از طریق یک مرحله از خواب، که در آن ارتباطات سیناپس انرژی را از سلول های عصبی آزاد می رود.شبکه های عصبی است و سپس با طراحی دیوار تصادفی جدید، که با استفاده از شبکه عصبی تجدید در تلاش برای طراحی دیوار بهتر فکر می کنم از دیدگاه های مختلف ارائه شده است. این فرایند نتیجه گیری پس از طراحی بهتر است پس از چند فکر و خواب چرخه را کشف کردند.
این فرایند به مراتب موثر تر از الگوریتم های جستجوی به حداقل رساندن جایگزین، مانند گرادیان مزدوج، آنیل شبیه سازی شده، و یا روش های مونت کارلو. گرادیان مزدوج فرود در دره در فضای جستجو را تا رسیدن به یک حداقل، نیاز به ارزیابی عملکرد های متعدد، و باید برای مکان های متعدد به صورت تصادفی در فضای جستجو، تکرار شود تا افزایش احتمال پیدا کردن یک راه حل خوب است. بازپخت شبیه سازی شده و دیگر تکنیک مونت کارلو نیز نیاز به ارزیابی عملکرد های متعدد، اما لازم نیست که تکرار شود. بازپخت شبیه سازی شده برای طراحی دیوار ناخن خاک به عنوان یک مقایسه انجام شده است. بازپخت شبیه سازی شده قادر به پیدا کردن نیمه راه حل خوب هم بعد از ارزیابی های طراحی ۱۲۰۰۰ دیوار، نیاز به حدود ۱۰ ساعت بود.شبکه های عصبی قادر به پیدا کردن یک راه حل خوب در هر زمان پس از ارزیابی های طراحی ۱۲۰ دیوار، در حدود ۱۰ دقیقه بود.
به عنوان مثال، طراحی دیوار های اخیر از یک طرح موجود به هزینه از $ ۳۵٫۴ M با حداقل ضریب ایمنی از ۱٫۳۵ توسط SNAP استفاده شد، ۹ پارامترهای مختلف. SNAP 127 طرح دیوار تولید شده از امکان ۶۷۲۰۰۰۰۰۰۰۰۰ برای به دست آوردن پیروزی های طراحی در کل هزینه $ ۲۱٫۰ M با حداقل ضریب ایمنی از ۱٫۳۵، در دقیقهی ۳۴٫ این صرفه جویی هزینه از $ ۱۴٫۴ M، و یا ۴۱٪، در همان عامل ایمنی بود. زمان مورد نیاز برای آموزش های عصبی شبکه، تجزیه و تحلیل منطقی و چرخه خواب ۱۵ دقیقه بود.زمان مورد نیاز برای جامع جستجوی همه ۶۷۲۰۰۰۰۰۰۰۰۰ طرح ممکن خواهد بود ۳۴۵٫۰۰۰ سال.
این تکنیک های هوش مصنوعی دارای طیف گسترده ای از برنامه های کاربردی فراتر از طراحی دیوار ناخن خاک. این موفقیت برای استفاده:
ورود اولین چیدن برای بررسی های ژئوفیزیک (حق ثبت اختراع، ۱۹۹۰)
برنامه اجرا در IBM 3090، به جای کار ۸۰ دکترا در
آموزش دیده با مشاهده کارشناسان انسان
زمین توپولوژی
نقطه LIDAR پردازش ابری
مورد استفاده در RockVision-3D (تصویر برداری زمین)، TRT (تونل)، CRSP-3D (rockfall) و SNAP-3 (ثبات شیب)
خب الحاق ورود به سیستم
مورد استفاده در RockVision-3D و TRT
جنگ های الکتریکی
پیاده سازی در قطعات نوری
وزن تجهیزات کاهش از سال ۲۰۰۰ پوند به ۲۰۰ £
درست ۱ میلیون سیگنال های بدون خطا و یا مثبت کاذب شناسایی
بهبود قابل توجهی، اجازه می دهد محل منابع قبل از تشخیص
نصب شده بر روی F-16
سیستم های ویژن برای جوشکاری رباتیک
در سیستم های MTS و رباتیک برای جوشکاری ۲ پا فولاد ضخیم نصب شده است، در هوا و در زیر آب
کار کرده در ۹۰٪ فساد تصویر را از دود و پاشش، outperforming اپراتور انسانی ۵۰٪
مدل سازی پارامتر جوش
مورد استفاده برای تعیین MIL-مشخصات برای نیروی دریایی ایالات متحده و مشخصات جوشکاری برای پل شیکاگو و آهن
تعیین فعلی، ولتاژ و سرعت مطلوب جوش
پیش بینی مهره نفوذ، کلاه، قدرت، کیفیت، و سرباره
مورد استفاده در برنامه های کاربردی جوشکاری رباتیک
مدل های پخت بتن در شافت های حفاری شده
مورد استفاده برای ساخت مدل های تجربی از حرارت هیدراتاسیون، توسعه، قدرت، سختی و انتقال حرارت در بتن مسلح
ارسال به انتشار FHWA: تغییرات سرعت در میان سوراخ نظرسنجی ورود صوتی
مدل های پخت بتن در شافت های حفاری شده
خودکار عملیات وسیله نقلیه معدن
سرعت کنترل و فرمان بر اساس سنسورهای دامنه مافوق صوت در گوشه و کنار خودرو و سطح بالا فرمان برای جهت
آموزش دیده با مشاهده اپراتور انسانی
آیا خودرو در سراسر در شرایط تنگ تبدیل
کارت اعتباری برنامه ریزی خدمات به مشتریان
تعداد پیش بینی شده از تماس مشتری در هر روز داده شده با استفاده از قبلی ۳ سال از داده ها، با استفاده از یک لپ تاپ در ۲۰ دقیقه (دقت ۹۰ درصد)
خارج انجام یک بخش آزمایشگاه ملی ایالات متحده، ریاضیات غیر خطی با استفاده از ۲۵ خورشید ایستگاه های کاری در حال اجرا ۶ ماه (۸۵٪ دقت)
پیش بینی آب و هوا
عملکرد منطبق از هواشناسان برای پیش بینی دما و بارش با توجه به اطلاعات آب و هوا قبل
پیش بینی نژاد اسب
زمان مسابقه پیش بینی اسب بر اساس سابقه قبلی، شرایط آهنگ، دروازه، و غیره
نوشتن موسیقی
آکورد گیتار تعیین شده از ملودی
NAP can obtain optimal to near-optimal soil nail wall designs in minutes. The program can adjust:
Wall
Height and batter angle
Nail
length
inclination angles
fan angles
horizontal and vertical spacing
cantilever distance
bar size
Drillhole diameter
Backslope cuts and downslope cuts
The user specifies valid ranges and resolutions for each parameter, as well as minimum factors of safety for seismic loading and static loading at each staging cut.
The VE design minimizes total cost while meeting all global stability safety factors. VE designs are obtained using the scientific method. The program:
Collects observations
Postulates causal relationships and proposes theories
Generates a hypothesis
Predicts results
Runs an experiment to acquire measurements
Compares predictions to the measurements
Re-evaluates theories, if necessary
Generates a new hypothesis
This process continues until proposed theories accurately predict any given design.
An artificial intelligence technique incorporating a specialized neural network is used to study observations, analyze parameters, propose theories, generate hypotheses, and predict results. The neural network simulates both the structure and function of the brain. It is trained on a set of observations (simulated wall designs) to predict results of future experiments (simulated wall designs) by analyzing causal relationships and interactions among parameters.
The neural network goes through a process of logical reasoning and thought to make an educated guess, or hypothesis, of the optimal wall design. The neural network predicts the price and minimum factor of safety of this wall design. The program then calculates a cost and a factor of safety for this wall design. If the neural network prediction is incorrect, the neural network re-analyzes parameter relationships to generate a new hypothesis for the best wall design. This process repeats until the neural network prediction of the hypothesis is correct.
The neural network then goes through a sleep stage, where the synapses connections release energy from the neurons. The neural network is then presented with a new random wall design, which the refreshed neural network uses in an attempt to think of a better wall design from a different perspective. The process concludes after no better design is discovered after several thought and sleep cycles.
This process is far more efficient than alternative minimization search algorithms, such as conjugate gradient, simulated annealing, or Monte Carlo techniques. Conjugate gradient descends through valleys in the search space until reaching a minimum, requiring numerous function evaluations, and must be repeated for numerous random locations in the search space to increase the probability of finding a good solution. Simulated annealing and other Monte Carlo techniques also require numerous function evaluations, but do not need to be repeated. Simulated annealing was implemented for soil nail wall design as a comparison. Simulated annealing was able to find a good solution half the time after 12,000 wall design evaluations, requiring about 10 hours. The neural network was able to find a good solution every time after 120 wall design evaluations, in about 10 minutes.
For example, a recent wall design from an existing project at a total cost of $35.4 M with a minimum factor of safety of 1.35 was analyzed by SNAP, varying 9 parameters. SNAP generated 127 wall designs out of a possible 672 billion to obtain a VE design at a total cost of $21.0 M with a minimum factor of safety of 1.35, in 34 minutes. This was a cost savings of $14.4 M, or 41%, at the same factor of safety. The time required for the neural network training, logical analysis, and sleep cycles was 15 minutes. The time required to exhaustively search all 672 billion possible designs would be 345,000 years.
This artificial intelligence technique has a wide range of applications beyond the design of soil nail walls. It has been successfully used for:
First arrival picking for geophysical surveys (patent, 1990)
Program implemented on IBM 3090, replacing work of 80 PhD’s
Trained by observing human experts
Terrain Topology
Lidar point cloud processing
Used in RockVision-3D (ground imaging), TRT (tunneling), CRSP-3D (rockfall), and SNAP-3 (slope stability)
Well log interpolation
Used in RockVision-3D and TRT
Electric warfare
Implemented in optical components
Decreased equipment weight from 2000 lbs to 200 lbs
Correctly identified 1 million signals with no errors or false positives
Significant improvement, allowing location of sources before detection
Installed on F-16
Vision systems for robotic welding
Installed on MTS systems and robotics for welding 2 foot thick steel, in air and under water
Worked in 90% image corruption from smoke and spatter, outperforming human operators by 50%
Weld parameter modeling
Used to determine mil-specs for US Navy and welding specs for Chicago Bridge and Iron
Determined optimal current, voltage, and weld speed
Predicted bead penetration, cap, strength, quality, and slag removal
Used in robotic welding applications
Concrete curing models in drilled shafts
Used to construct empirical models of heat of hydration, expansion, strength, stiffness, and heat transfer within reinforced concrete
Submitted as FHWA publication: Velocity Variations in Cross-hole Sonic Logging Surveys
Concrete curing models in drilled shafts
Automated mining vehicle operation
Controlled speed and steering based on ultrasonic range sensors at corners of vehicle and high level commands for direction
Trained by observing human operator
Could turn vehicle around in tight conditions
Credit card customer service scheduling
Predicted number of customer calls on any given day using previous 3 years of data, using a laptop in 20 minutes (90% accuracy)
Out-performed a US national lab, non-linear mathematics division, using 25 Sun workstations running 6 months (85% accuracy)
Weather prediction
Matched performance of meteorologists for prediction of temperature and precipitation given previous weather data
Horse race prediction
Predicted race time of a horse based on previous history, track conditions, gate, etc.
Writing music
Determined guitar chords from melody
سایت سازنده
تیم مهندسی معکوس crack4all.
جهت سفارش دانلود کرک از طریق ثبت درخواست و یا تماس با شرکت اقدام فرمایید